uos-machine-learning
Text to Speech 딥보이스 3 돌려만보기 - Deep Voice 3 (Pytorch) 본문
오늘은 Text to Speech 딥 보이스 코드를 실행해보고 뜯어보는 시간을 가지겠습니다. 만들어진 오디오 샘플은 아래링크에서 확인가능하다고 합니다. 시작하기 전에 아래 코드로 먼저 설치해주세요. 다만 위 깃허브는 아웃풋을 실제 음성으로 만들 때 쓰는 World 알고리즘이 생략되어 있습니다. World 알고리즘이 구현된 코드가 궁금하시다면 링크로 들어가시면 됩니다.
0. Installation
git clone https://github.com/r9y9/deepvoice3_pytorch && cd deepvoice3_pytorch
pip install -e ".[bin]"
1. Getting Started
1-0. Preset Parameters
이 코드에서는 많은 파라미터를 사용할 수 있습니다. 공개된 데이터셋에 맞춰 적절한 json 파라미터 파일을 제공하고 있습니다. 파라미터 json 파일은 presets 디렉토리에서 확인할 수 있습니다. 전체 TTS 합성과정 다음과 같은 과정으로 진행됩니다.
- preprocess.py
- train.py
- synthesis.py
위 3가지 스크립트를 거치면 합성된 오디오 파일을 html에 붙여서 만들어 줍니다. 우선 데이터셋 다운로드 부터 해보겠습니다.
1-1. Download dataset
-
더보기LJSpeech (en): https://keithito.com/LJ-Speech-Dataset/
각각의 링크에서 다운로드 받을 수 있습니다. 이 때 파일 구조는 다음과 같습니다.

저 같은 경우에는 github 안에 data 디렉토리를 만들고 그 안에 dataset을 다운로드 받았습니다. metadata.csv를 열면 wavs 폴더에 있는 음성 파일의 이름과 그 파일에서 나오는 텍스트가 쌍으로 존재합니다.
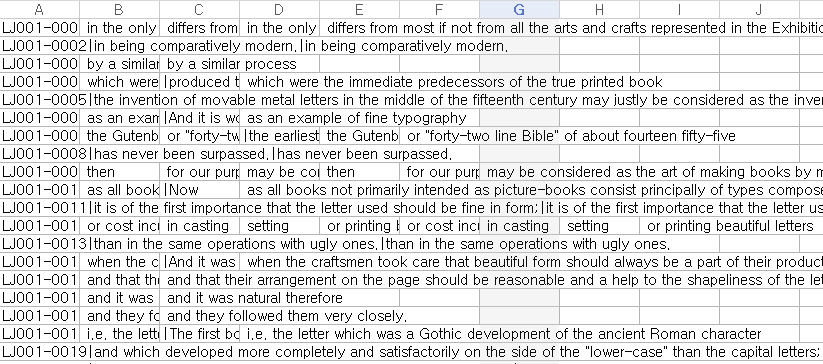
1-2. Preprocessing
아래 스크립트를 사용하면 데이터 파일에 있는 .wav를 전처리하여 mel-spectogram을 넘파이 배열로 바꿔 명시한 output 디렉토리에 새로 저장을 해줍니다.
python preprocess.py ${dataset_name} ${dataset_path} ${out_dir} --preset=<json>
Supported ${dataset_name}s are:
- ljspeech (en, single speaker)
- vctk (en, multi-speaker)
- jsut (jp, single speaker)
- nikl_m (ko, multi-speaker)
- nikl_s (ko, single speaker)
다운 받은 데이터 셋 중에 하나를 선택하여 실행을 하면 됩니다. 아래는 ljspeech 데이터셋을 사용하여 실행하는 명령어 예시입니다.
python preprocess.py --preset=presets/deepvoice3_ljspeech.json ljspeech ./data/LJSpeech-1.0/ ./data/ljspeech
그러면 아래와 같이 .npy로 전처리가 완료된 데이터가 만들어집니다.
1-3. Training
아래 스크립트로 학습을 진행을 하면 됩니다.
python train.py --data-root=${data-root} --preset=<json> --hparams="parameters you may want to override"
만약 ljspeech 데이터셋을 사용한다면 아래 스크립트가 그 예시가 될 수 있습니다.
python train.py --preset=presets/deepvoice3_ljspeech.json --data-root=./data/ljspeech/
default 로 10000 step 마다 checkpoint 폴더에 모델 체크포인트 (.pth)와 alignment (.png)를 저장합니다.
결과물 예시
오류났던 부분
이런 경우에는 Searched in 에 있는 디렉토리에 nltk_data 폴더를 새로 생성하고 그 아래 corpora 라는 이름의 폴더 생성후 아래 링크에서 cmudict.zip 을 다운 후 압축해제 하면 됩니다.
http://www.nltk.org/nltk_data/
1-4. Monitor with Tensorboard
로그들은 ./log 폴더에서 확인할 수 있습니다.
tensorboard --logdir=log
Scalar
loss에 대한 전반적인 모니터링을 할 수 있습니다.
그 중에 예시를 살펴보면 점점 수렴하는 모습을 확인할 수 있습니다.
![]() |
![]() |
Images
Images 배너에서는 Target 음성의 Mel / Linear spectogram과 Prediction 스펙토그램을 비교해볼 수 있습니다.
Predicted![]() |
Target![]() |
Audio
오디오 배너에서는 만들어진 샘플을 텐서보드에서 실제로 들어볼 수 있습니다.
1-5. Synthesize from a checkpoint
synthesis.py 에서 .txt 파일로부터 오디오 시그널을 합성하고 이를 html 파일로 저장할 수도 있습니다. 합성을 하면 wav파일이 output_dir에 저장됩니다.
python synthesis.py ${checkpoint_path} ${text_list.txt} ${output_dir} --preset=<json>
실행했던 명령어 예시입니다.
python synthesis.py ./checkpoint_step000020000.pth test_list.txt ./output_dir --preset=presets/deepvoice3_ljspeech.json
Example test_list.txt
His palms are sweaty, knees weak, arms are heavy There's vomit on his sweater already, mom's spaghetti He's nervous, but on the surface he looks calm and ready To drop bombs, but he keeps on forgettin' What he wrote down, the whole crowd goes so loud |
2. 더 공부해볼 것
- Custom Dataset으로 돌려보기
- TTS Text 입력하면 음성으로 들려주는 웹 사이트 만들어 보기
'딥러닝' 카테고리의 다른 글
Yolo v3 논문 리뷰 (0) | 2019.09.18 |
---|---|
파이썬 라이브러리 소개 - imgaug (1) | 2019.09.12 |
CycleGAN Implementataion 코드에 Wasserstein loss 추가하기(Pytorch) (0) | 2019.09.08 |
Tensorflow 2.0 Neural Style Transfer 튜토리얼 (5) | 2019.05.14 |
Image Super Resolution Evaluation Metric 케라스 구현 (0) | 2019.05.11 |